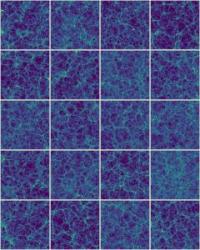
Cutting-edge research in modern cosmology demands accurate theoretical predictions based on physical models and their detailed validation against observational data. Statistics plays a crucial role in the validation of physical models. It enables determination of the most likely physical model amongst the zoo of plausible models, given the observational data. In light of recent large-scale observational programs, modern cosmology has entered the big-data science era. Cosmology is now an interdisciplinary research area, which requires cosmologists at the Kavli IPMU to work together with statisticians and computer scientists.
The need for statistical methods for the progress of modern cosmology is clear from the probabilistic nature of the Universe. According to the concordance model of cosmic structure formation, all the inhomogeneities presently seen in the Universe, including stars, galaxies, galaxy clusters and the large scale cosmic web, have their origin in the tiny quantum fluctuations that arose during the inflationary period in the very early Universe. These tiny fluctuations, as seen imprinted on the cosmic microwave background radiation right after cosmic recombination, grow by gravitational instability, eventually forming the present rich structure in the Universe.
However, the quantum fluctuations which form the initial conditions are intrinsically probabilistic. Hence, the exact spacetime location of any astronomical object cannot be exactly predicted, even if we completely understand the physical laws that govern the Universe. The observed cosmic structures are merely one possible random sample drawn from an unknown probability distribution. Therefore, model comparisons to data and subsequent model inference, necessitates the use of summary statistics extracted in a similar manner from both data and theoretical models. Moreover, statistics is needed in a wider range of topics in astronomy to account for measurement errors and incomplete data. To put in simple words, statistics is essential for the optimal use of astronomical data sets to unveil the physics that governs our Universe.
Researchers at the Kavli IPMU make use of state-of-the-art techniques developed in statistics to tackle these problems. Although we have a single observable Universe, we can perform statistical studies by generating a large number of mock universes in our computers. We conduct simulations that generate big data which surpass the data volume generated by observations. We need fast and accurate theoretical predictions of summary statistics for model comparison. We apply statistical and machine learning techniques, using the mock universes as training data, to achieve such efficient theoretical predictions. We are developing a Bayesian inference pipeline to bracket the most likely cosmological model and its parameters through detailed comparison with observational data. Our research also includes the development of the theory of advanced summary statistics, which go beyond the conventional two-point statistics commonly used in cosmology, to understand their information content and to convert them into practical tools. Our research also deals with other areas where statistics is important, e.g., the recovery of three-dimensional cosmic structures from the two-dimensional weak gravitational lensing data based on sparse modeling techniques. We are also applying machine learning to efficiently pick up likely candidates of transient astronomical phenomena, such as supernovae or moving objects such as asteroids, from massive imaging data. The goal is to automatically classify such phenomena, a work which has previously required human eyes on a case-by-case basis. These efforts are gradually paying off in the statistical analyses of real data obtained by Subaru HSC. (Last update: 2018/05/08)
Link
Related Observational Project: Hyper Suprime-Cam (HSC)
Members
- Joaquin Andres Armijo Torres
- Linda Blot
- Neal Dalal
- Andrew Eberhardt
- Tomohiro Fujita
- Elisa Gouvea Mauricio Ferreira
- Anamaria Hell
- Benjamin Horowitz
- Baptiste Jost
- Clement Leloup
- Jia Liu
- Kevin McCarthy
- Hironao Miyatake
- Marta Monelli
- Anupreeta Sadashiv More
- Surhud More
- Kentaro Nagamine
- Toshiya Namikawa
- Nhat Minh Nguyen
- Takahiro Nishimichi
- Yasunori Nomura
- Masamune Oguri
- Sebastien Peirani
- Shi Pi
- Yuki Sakurai
- Jingjing Shi
- Xue Song
- Tomomi Sunayama
- Masahiro Takada
- Volodymyr Takhistov
- Atsushi Taruya
- Leander Thiele
- Edwin Turner
- Fumio Uchida
- Marcus Werner
- Naoki Yasuda
- Jun'ichi Yokoyama
- Naoki Yoshida
- Jiaxi Yu